AI-Driven Robotic Systems Revolutionize Drug Discovery in Next-Gen Laboratories
AI-Driven Robotic Systems Revolutionize Drug Discovery in Next-Gen Laboratories - AI algorithms slash drug discovery timelines from years to months
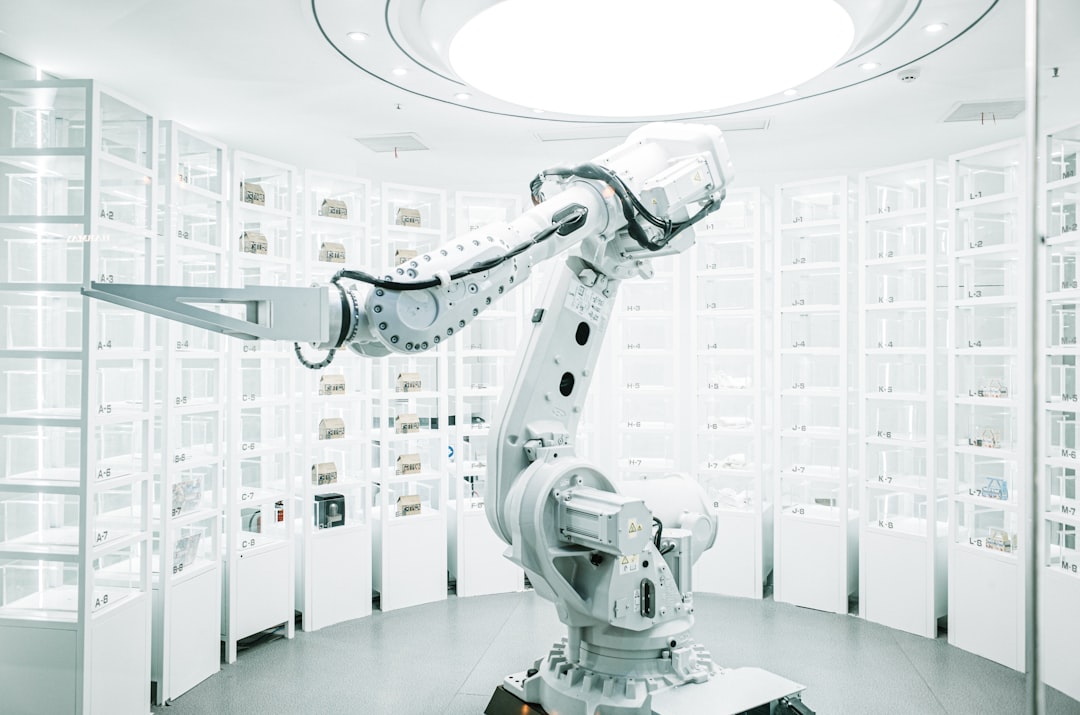
Artificial intelligence algorithms are fundamentally altering the pace of drug discovery, compressing what once took years into a matter of months. The power of advanced machine learning is being harnessed to accelerate drug development, enabling rapid analysis of vast datasets and quicker identification of promising drug candidates. This shift from initial AI concepts to practical applications within the drug discovery field signifies a crucial maturation of the technology. AI's ability to autonomously analyze data and make decisions has started to transform the pharmaceutical sector. It's noteworthy that the growing sophistication of AI-powered robots within next-generation laboratories complements these advancements, making drug discovery processes even more efficient. While this progress is undeniable, it is crucial to remember that challenges still persist in fully integrating and optimizing these new AI tools within the complex field of pharmaceutical research.
It's remarkable how AI algorithms are dramatically shrinking the timeline for drug discovery, potentially cutting years-long processes down to mere months. This is largely due to the power of advanced machine learning, which can sift through enormous datasets – encompassing genomics, proteomics, and vast chemical libraries – to uncover intricate patterns and interactions human researchers might miss. These algorithms can predict how molecules will interact with targets in the body, improving the accuracy of lead optimization and minimizing the need for costly, iterative experimentation.
One aspect that's caught my attention is the potential of AI to automate many repetitive tasks within drug discovery, freeing scientists to focus on more conceptual challenges and novel solutions. There's also the possibility of leveraging AI to identify potential "drug repurposing" candidates. Essentially, we can potentially mine existing medications for new applications, significantly speeding up treatment development for various ailments. Furthermore, with enhanced computational models, drug interactions can be simulated at the molecular level, potentially paving the way for designing medications that are both more effective and less prone to side effects.
The ability to share data and findings through collaborative AI platforms could accelerate the collective understanding of complex diseases and accelerate the development of new treatments. Additionally, the predictive capabilities of AI allow for early-stage forecasting of a drug candidate's potential success based on historical data. This could be transformative for streamlining decision-making in drug development. It's also intriguing how AI is contributing to the field of personalized medicine, where treatment strategies are tailored to an individual's genetic makeup, thanks to sophisticated algorithmic analyses.
While the benefits of AI in drug discovery are exciting, it's crucial to remember that we need to carefully scrutinize the results of these AI algorithms. Data bias can creep into these systems, and it's essential to have human oversight to ensure the reliability and applicability of AI-derived insights in real-world clinical settings. This critical validation step will be crucial to safeguard against potential flaws and to maintain trust in the development of new, potentially lifesaving treatments.
AI-Driven Robotic Systems Revolutionize Drug Discovery in Next-Gen Laboratories - Insilico Medicine unveils fully automated AI-driven robotics laboratory
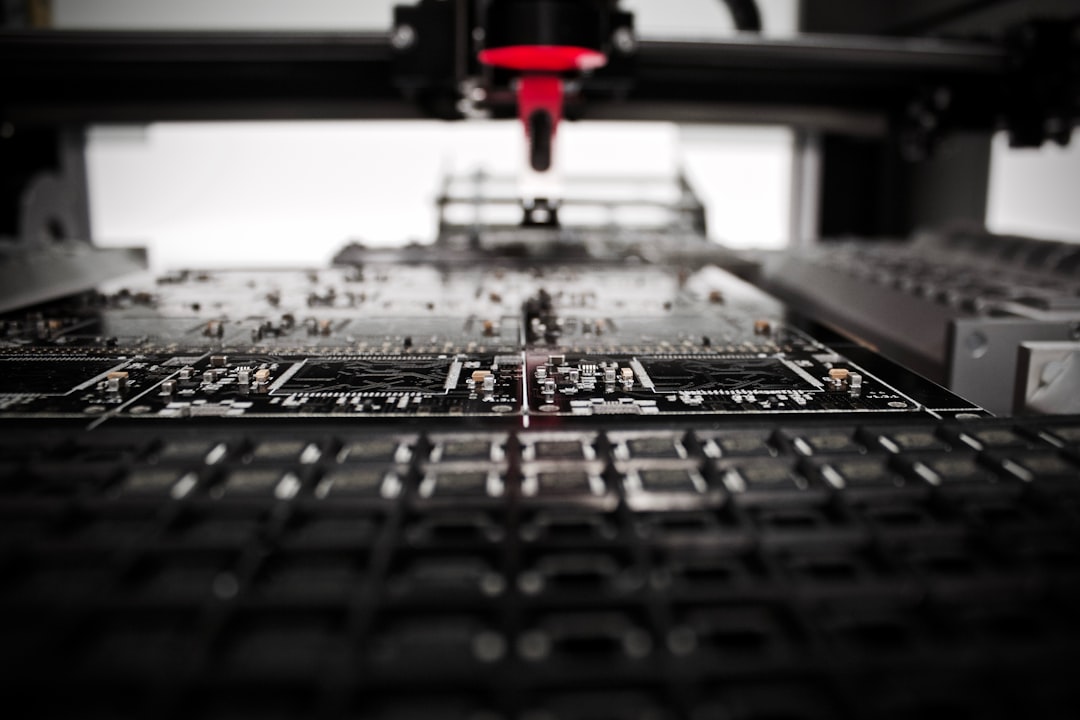
Insilico Medicine has unveiled a fully automated, AI-powered robotics laboratory called "Life Star," anticipated to be fully operational within the current year. This laboratory, which has been in development for the past two years, is central to Insilico's comprehensive AI-driven drug discovery platform. It's designed to handle a range of tasks, including identifying potential drug targets, screening compounds, developing personalized medicine approaches, and conducting translational research. The lab's remote operation capability facilitates streamlined communication and collaboration among researchers across various experiments. By integrating Insilico's PharmaAI platform, the lab functions as a closed-loop system that uses AI to make more informed decisions throughout the drug discovery process. Ultimately, this lab's goal is to expedite drug discovery and increase the chances of successfully developing new therapies. It’s a noteworthy step towards more automated and intelligent drug discovery processes, but whether it can truly solve the complexities of drug development remains to be seen. The success hinges on the ability to overcome integration challenges within the existing pharmaceutical research environment.
Insilico Medicine has unveiled a fully automated AI-driven robotics laboratory, dubbed "Life Star," which is expected to be fully operational this year. It's been in development for the past two years, and is a core component of Insilico's comprehensive AI-driven drug discovery platform. The lab's launch ceremony took place in Suzhou BioBAY Industrial Park late last year.
This facility will automate various stages of the drug discovery pipeline, including target identification, compound screening, the development of personalized medicine approaches, and translational research. Ultimately, it aims to accelerate the entire drug discovery process and improve the success rate of developing new therapeutic agents.
Essentially, the laboratory operates as a closed-loop system that seamlessly integrates Insilico's PharmaAI platform. This integration is designed to enhance decision-making within the drug discovery process, allowing for more informed choices and potentially reducing the number of failed experiments.
The laboratory is designed for remote operation, which is expected to enhance efficiency and collaboration among researchers across diverse teams and experiments. This launch is part of Insilico's broader strategy to revolutionize drug discovery practices within next-generation laboratory environments.
The development of this robotics lab certainly strengthens Insilico Medicine's capabilities in AI-driven drug development, potentially increasing overall R&D efficiency. It's a noteworthy step towards automating and enhancing the intelligence of drug discovery processes by leveraging advanced AI and robotics. While promising, it's crucial to recognize that the successful integration of AI into such a complex field remains a considerable challenge.
This automated laboratory represents a considerable change in the way drug research is conducted. It can handle various tasks, from initial screening to advanced bioinformatics analysis, which were traditionally performed by a variety of specialized machines in separate laboratory environments. It's intriguing to note that this automated environment is capable of learning from past experimental outcomes. The system continuously adjusts protocols based on prior results, progressively improving efficiency and accuracy over time.
The lab's ability to operate around the clock vastly increases the throughput of research compared to conventional laboratories. This continuous workflow offers a significant advantage in the drug discovery process. An unexpected benefit of this automation is the laboratory's ability to provide real-time feedback and correction during experiments. Through this process, failed experiments can be identified quickly and the knowledge used to adjust future iterations.
Further, the laboratory is capable of processing enormous amounts of data, on the order of petabytes, allowing for intricate drug interaction modeling and simulation at an unprecedented scale. The robotic systems within the laboratory are also designed to communicate with each other. They can autonomously share data and insights, which could potentially lead to discoveries that would be overlooked by a single robotic unit or individual researcher.
It is also noteworthy that this laboratory greatly reduces the need for conventional chemical synthesis which is often time-consuming and resource-intensive. This aspect, focusing on virtual experimentation, has the potential to transform the early-stage drug discovery process. Interestingly, the individual robots in the lab incorporate machine learning capabilities allowing them to adapt and modify their configurations based on ongoing research. This level of flexibility is rarely found in conventional laboratory environments.
The integration of these robotics systems also compels us to ponder the future of the drug discovery workforce. As AI and automation become more sophisticated, the balance between human innovation and machine-driven efficiency continues to evolve. This raises questions about the role of scientists and engineers in a rapidly changing pharmaceutical industry.
AI-Driven Robotic Systems Revolutionize Drug Discovery in Next-Gen Laboratories - Machine learning systems autonomously analyze vast chemical databases
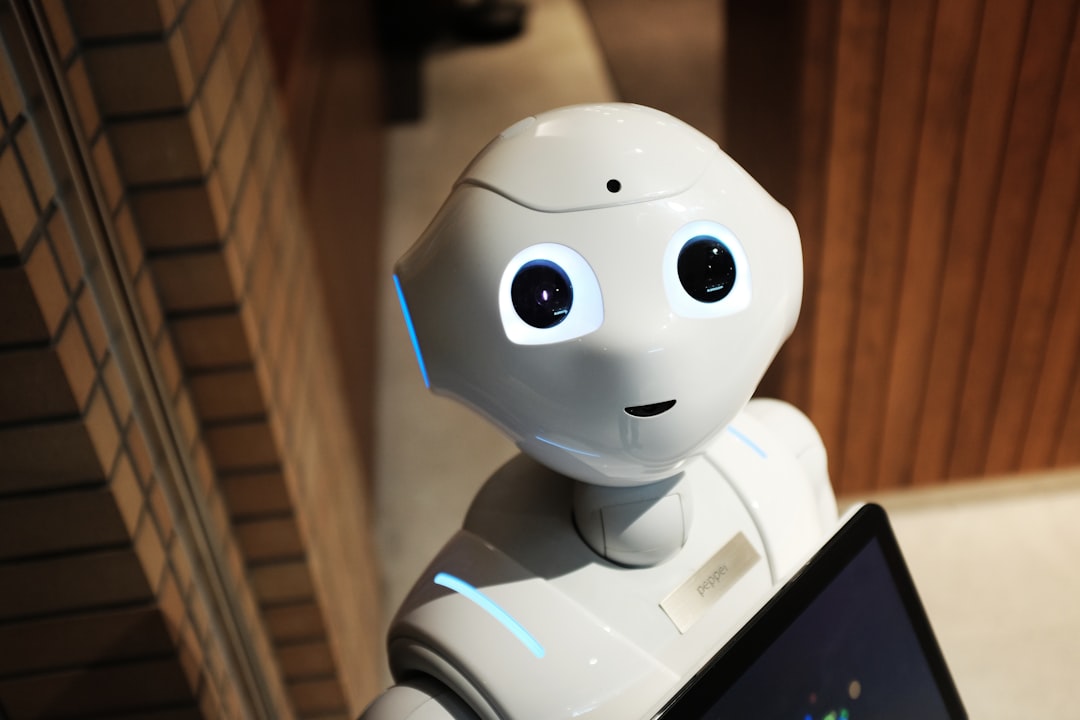
Machine learning systems are now capable of autonomously sifting through massive chemical databases, significantly enhancing the speed and effectiveness of data analysis. These systems can identify complex patterns and relationships between molecular structures and their characteristics, revealing insights that would be difficult for humans to uncover manually. This automated analysis, coupled with AI-driven robotic systems in advanced laboratories, facilitates a more streamlined approach to tackling complex chemical challenges in drug discovery. While these technologies hold immense promise, it's important to acknowledge the ongoing need to ensure that the data these systems produce is reliable and free from bias. Human oversight is crucial for validating AI-generated insights and guaranteeing their applicability in real-world settings, particularly when dealing with the development of new drugs. The successful integration of AI into this complex area of research will continue to depend on addressing these challenges.
Machine learning systems are now able to autonomously sift through massive chemical databases, which house millions of compounds. This capability enables incredibly fast identification of potential new drug candidates, a process that would take human researchers significantly longer. It's fascinating how these systems can spot correlations between molecular structures and their biological effects, often revealing previously unknown ways existing drugs work. This kind of insight is hard to uncover using traditional techniques.
Furthermore, advanced predictive modeling techniques within these systems allow for simulations of how even minor tweaks to a molecule's structure can influence its properties. This allows chemists to fine-tune lead compounds before they even begin lab testing, leading to a more efficient workflow. The machine learning algorithms aren't just trained on chemical data; they can also incorporate information from clinical trials. This cross-referencing enhances their ability to predict a drug's effectiveness and safety based on past performance, which is invaluable.
I find the collaborative aspect of these machine learning platforms particularly interesting. They allow researchers from various fields to contribute and improve the algorithms, which often results in novel approaches to drug discovery that transcend typical departmental boundaries. By taking over much of the data analysis, these systems can reduce the chance of human error in interpreting vast datasets. This is vital in pharmaceutical research, where mistakes can have major consequences.
Intriguingly, some machine learning models can even generate completely new chemical structures, employing principles of generative design to create molecules that are potentially more likely to be therapeutically effective. These systems also exhibit continuous learning capabilities; they refine their predictive abilities as new data becomes available. This makes them incredibly adaptable and responsive to ongoing research developments.
The scalability of machine learning is another key advantage. It enables real-time analysis of experimental findings, significantly reducing the iterative design and testing phases that often create bottlenecks in traditional drug discovery processes. While these capabilities are promising, we must be mindful of potential risks associated with relying on machine learning systems. Data biases can creep in, potentially affecting the reliability of conclusions drawn from these technologies. Therefore, rigorous validation and human oversight are critical to ensure the credibility of insights derived from these systems. This careful scrutiny is vital to maintain trust in the development of new, possibly life-saving treatments.
AI-Driven Robotic Systems Revolutionize Drug Discovery in Next-Gen Laboratories - AI-powered platforms identify novel drug targets for untreatable diseases
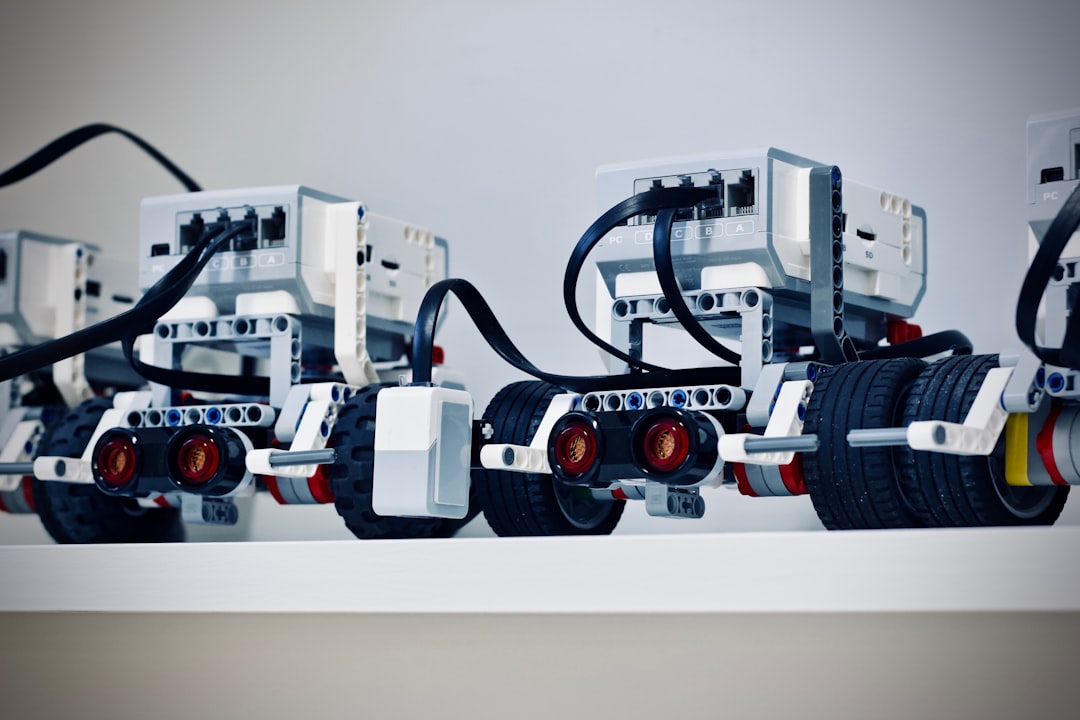
AI-powered platforms are revolutionizing drug discovery by identifying novel drug targets for diseases previously considered untreatable. These platforms leverage sophisticated machine learning to analyze massive amounts of genetic and protein data, uncovering hidden connections within disease pathways that might evade human researchers. This is particularly helpful for diseases where traditional treatments have proven ineffective. AI's ability to independently analyze and interpret complex datasets boosts the prospect of developing therapies tailored to individual patients, a potential game-changer in drug development. Despite these exciting possibilities, careful evaluation and oversight are crucial to address potential issues and biases inherent in AI-powered research, particularly when developing new medications.
AI-driven platforms are significantly accelerating the discovery of novel drug targets, particularly for diseases that have previously been resistant to treatment. This speed-up, from years to potentially months, is a game-changer for the development of new therapies. One way this is achieved is through the ability of AI to analyze a much broader range of data, including genomics, transcriptomics, and proteomics. This wider view allows for the identification of intricate biological networks that might otherwise be missed, potentially leading to entirely new therapeutic approaches.
Furthermore, the advanced algorithms used within these platforms can simulate how molecules interact within the body. This predictive power allows researchers to anticipate the impact of design changes on drug efficacy and side effects. This insight can streamline the process of refining potential drug candidates, optimizing them before they even enter the laboratory testing phase. It's noteworthy that some AI systems are now using a process called reinforcement learning to explore the vast chemical landscape and automatically find new drug targets. This automated approach reduces the reliance on traditional, hypothesis-driven research which can be a much slower and less efficient process.
Another intriguing application is the ability to identify existing drugs that might be effective against diseases that were previously considered untreatable. This "drug repurposing" approach can dramatically cut the time needed to develop a new treatment. Researchers are actively developing ways to combat inherent bias in training data, making the insights gleaned from AI-powered systems more reliable. By cross-referencing diverse datasets, these systems aim to provide more robust and less skewed assessments of potential drug targets.
AI systems are also becoming more adaptable, capable of modifying their learning algorithms in response to new data in real-time. This means that the AI remains up-to-date with the latest research, ensuring predictive capabilities stay relevant. One of the exciting aspects of AI in drug discovery is the potential for increased collaboration among scientists from diverse fields. AI platforms can serve as central hubs for sharing data and insights, potentially fostering innovative solutions to challenging biochemical problems.
Perhaps one of the most important improvements is the ability to incorporate data from previous clinical trials. By training AI on the successes and failures of past drug development efforts, we can generate predictions that are grounded in real-world evidence of a drug's efficacy and safety profile. Moreover, the sheer scale of data that these systems can handle is staggering. The capability to analyze petabytes of information simultaneously greatly enhances the efficiency of drug discovery, surpassing the analytical capacity of traditional laboratories which are still limited by the scale of human-driven data processing. The speed and efficiency gains from AI-powered drug discovery represent a significant step forward, especially for rare or neglected diseases where time-sensitive treatment options are urgently needed. While this field is progressing rapidly, ongoing research is necessary to refine these methods and mitigate potential challenges.
AI-Driven Robotic Systems Revolutionize Drug Discovery in Next-Gen Laboratories - Robotic systems perform high-throughput screening of millions of compounds
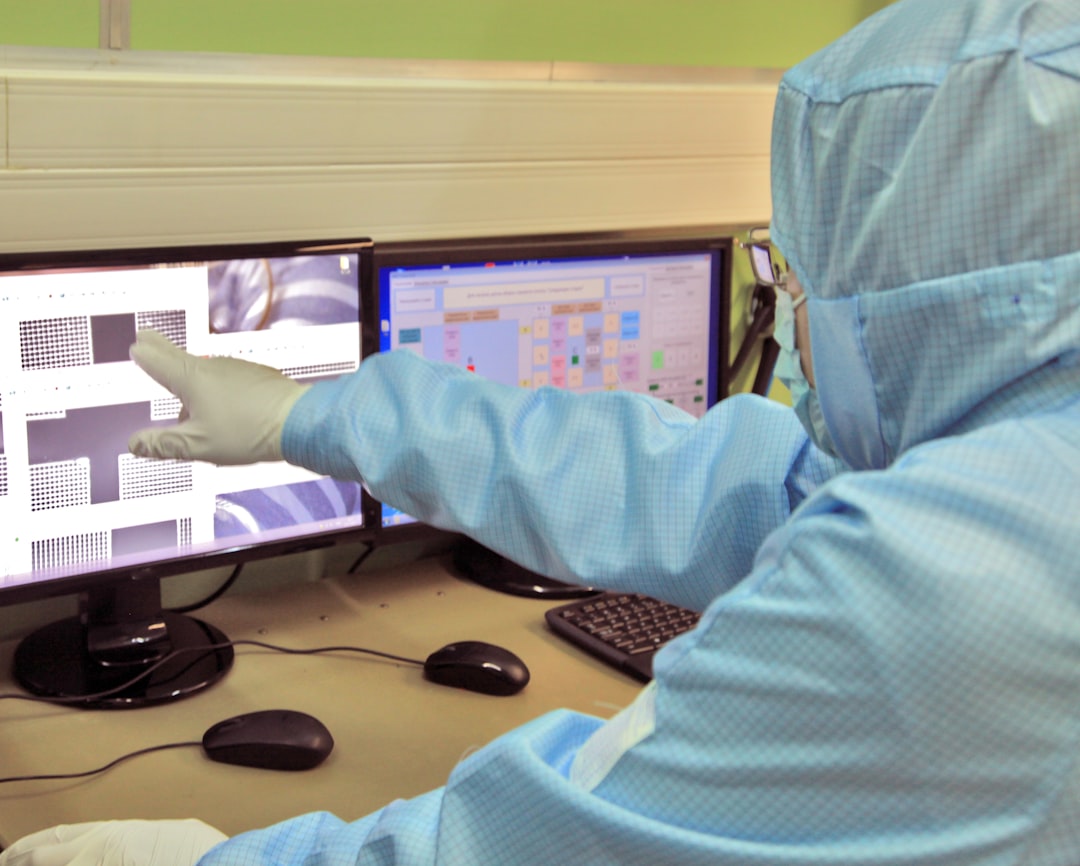
Robotic systems play a crucial role in accelerating drug discovery by enabling high-throughput screening (HTS) of massive compound libraries, often containing millions of potential drug candidates. This automated process has become increasingly vital in recent years for efficiently identifying compounds with promising biological activity. Typically, these compounds are stored and tested in microtitre plates, allowing robots to rapidly screen large numbers of them under various conditions. The integration of AI further refines this process, improving both the accuracy and overall efficiency of HTS compared to traditional methods. While these robotic systems offer undeniable advantages, drug development remains a highly complex field. This complexity demands careful consideration of the results generated by AI, ensuring reliability and mitigating the risk of biases within the algorithms used in the screening process. The continuing need for human oversight to validate findings from these AI-driven systems is key to maintain the integrity of drug discovery and ensure future safety and efficacy of new medications.
Robotic systems are increasingly being employed to accelerate the process of drug discovery by performing high-throughput screening on vast libraries of chemical compounds. Traditionally, screening millions of potential drug candidates was a painstaking and time-consuming process, potentially taking years to complete. However, robotic systems can now sift through these massive chemical libraries in a matter of days, significantly expediting the identification of promising candidates.
One of the key advantages of these robotic systems is their ability to automate many of the repetitive tasks involved in screening, such as liquid handling and sample preparation. This not only reduces the potential for human error but also ensures consistency across multiple experiments. Furthermore, some of these advanced systems can screen hundreds of thousands of compounds in a single day. The ability to assess such a massive number of compounds quickly is crucial when searching for novel therapeutic agents.
These robotic systems are often integrated with sophisticated data management systems, enabling real-time analysis and feedback during experiments. This continuous data flow can be valuable for refining the drug synthesis processes. It's fascinating how the integration of artificial intelligence and robotics allows the screening protocols to adapt and learn from experimental outcomes. As the system is fed more data, it continually refines its approach, leading to increasingly accurate predictions about the efficacy of different compounds.
Another notable aspect of these next-generation laboratory systems is the potential for remote experimentation. Researchers can now initiate, monitor, and analyze experiments from anywhere globally, which has fostered enhanced collaboration across different research teams. Although human expertise is still crucial in drug discovery, the increased automation of high-throughput screenings allows scientists to focus on more complex research challenges that require creative solutions. These robotic systems are designed to function with minimal human supervision, particularly during the screening phase.
Interestingly, many robotic platforms can evaluate multiple biological parameters simultaneously, offering a comprehensive view of how compounds interact within complex biological systems. Instead of a traditional approach of studying a single factor at a time, the systems provide a much more detailed understanding of how various biological factors are impacted by different compounds. The incorporation of AI further allows these systems to dynamically adapt their screening strategy during an experiment based on the initial results. This adaptive approach means that the protocols are not rigid, and can optimize themselves continuously without manual intervention for each experiment.
It's important to note that these technological advancements have the potential to reduce the overall costs associated with drug discovery. By decreasing the time and resources required to identify and validate new compounds, the entire drug development process becomes more cost-effective. While these automated systems are undeniably helpful in the drug discovery pipeline, it is important to continue critical evaluation and cautious application of these technologies to minimize unintended bias and maximize the reliable generation of knowledge.
AI-Driven Robotic Systems Revolutionize Drug Discovery in Next-Gen Laboratories - AI augments human researchers in designing more effective therapeutics
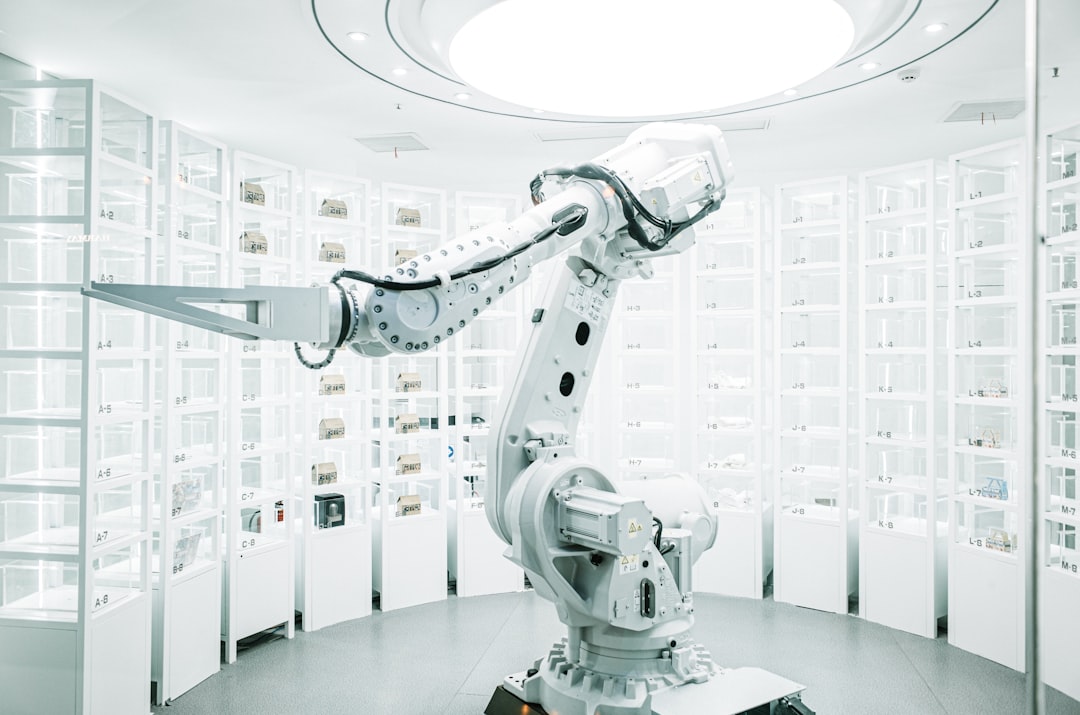
Artificial intelligence is increasingly playing a pivotal role in the creation of more effective therapies, working in tandem with human researchers rather than substituting them. AI's advanced computational abilities allow it to analyze massive datasets and explore the complexities of biological systems, significantly shortening the traditional drug development timeline. This synergy facilitates the identification of promising drug targets and enables the simulation of intricate drug interactions at a molecular level, leading to more accurate predictions of therapeutic outcomes. While this integration of AI promises substantial benefits, it is crucial to maintain a critical perspective. Human oversight remains essential to ensure that AI-generated insights are not skewed by inherent biases in the data, ultimately guaranteeing their reliability and clinical relevance. The process of integrating AI seamlessly into the therapeutic design process is fraught with complexities, but the potential for discovering novel treatments and advancing medicine is undeniably compelling.
AI is proving to be a valuable collaborator in the design of more effective therapies. It can uncover connections between molecular features and therapeutic actions that might otherwise be missed by traditional methods. This is particularly helpful when dealing with complex diseases, such as cancer, where highly targeted treatments are often needed. For instance, AI could illuminate pathways that haven't been explored before, leading to innovative treatment options.
Beyond just designing new drugs, AI is assisting in refining existing ones. By leveraging predictive modeling, we can fine-tune a drug's molecular structure before even starting lab tests. This optimization process could significantly speed up the path to clinical use and potentially reduce side effects.
One impressive ability of AI is its capacity to efficiently search through the entirety of published scientific literature. Humans would take ages to do this manually, but AI can find patterns and novel drug interactions or mechanisms of action in a very short time. This reveals new paths for drug development we might not have otherwise found.
AI platforms using reinforcement learning can automatically explore chemical space, which is incredibly vast. They actively seek out and prioritize drug targets that might have been overlooked with traditional hypothesis-driven approaches. This method has the potential to be quicker and more adaptable to changing needs in drug discovery.
However, AI can also generate entirely new drug molecules from scratch using generative design. This ability raises serious questions about the potential safety and ethical implications of creating completely novel drugs. We'll need thoughtful regulatory frameworks to guide this type of innovation and ensure safety throughout the process.
AI-powered platforms promote collaboration between different research disciplines. Diverse perspectives and expertise lead to a more comprehensive and robust drug discovery process. It's like creating a "hub" where researchers can share information and findings that wouldn't normally cross paths.
One of AI's unique capabilities is the ability to make real-time adjustments to experimental conditions during a drug development project. This dynamic approach offers a much quicker route to identifying failed attempts and helps improve the learning process through trial and error, a feature that wasn't present in older techniques.
The capacity of AI to analyze huge amounts of data allows us to combine different datasets – genetic, protein, and chemical – which can help uncover unexpected relationships. This type of comprehensive analysis leads to more effective drug development strategies by considering a wide range of factors.
An interesting consequence of AI’s integration into drug research is a reduction in the reliance on animal and human testing in the initial stages. AI can perform “in silico” experiments, simulating drug interactions and their effects. This shift could establish a new era in ethical drug development.
As AI systems learn from data as experiments are happening, they can improve the predictions of drug interactions and effectiveness. This learning loop allows them to autonomously develop and test hypotheses that can move research beyond conventional methods. This suggests a future where AI plays a more integral role in guiding the direction of discovery itself.
More Posts from agustin-otegui.com: